In this article, you’ll learn:
A few years back, "artificial intelligence" sounded like something straight out of sci-fi books and movies. Now, it’s everywhere—almost to the point of being overhyped. We’ll be honest, sometimes even we get a little tired of seeing AI pop up all over the place. But here’s the thing: we’re part of the tech world at Pics.io, and we’ve been excited about AI long before it went mainstream. When those first neural networks and AI bots hit the scene, we couldn’t resist testing them out. Our immediate thought was, "How can we use this to improve DAM?"
Think about it—DAM already saves each employee at least 10 hours a month that they’d normally spend searching for assets. But even the best DAM system requires a fair amount of manual work. That’s where AI comes in. Over the past few years, we’ve introduced AI features to take the heavy lifting out of asset management, making it not only more efficient but also a whole lot more satisfying.
In this post, we are excited to share how AI is making life easier for digital asset management users, and how we’ve been integrating it into Pics.io to make your journey smoother and more enjoyable.
Benefits of AI in Digital Asset Management
So, what exactly does AI bring to the world of digital asset management (DAM)?
In practical terms, AI in DAM can automate time-consuming processes like metadata tagging, asset descriptions, and even image recognition. Many Pics.io DAM users manage thousands of images, videos, and documents, which are often scattered across their systems. Without proper metadata—such as keywords, descriptions, or custom fields—finding exactly what they need can feel like playing an endless game of hide-and-seek.
That’s where AI comes to the rescue. At Pics.io, we’ve harnessed the power of AI to not only create metadata in seconds but also make searching for assets as smooth and practical as possible. We regularly engage with our users to gather honest feedback, and it’s this input that helps us continuously improve our DAM platform. We’ve even heard stories where teams had to assign someone just to manually add metadata to every single asset in a massive, chaotic library before they could fully benefit from the DAM. Ridiculous, right? That’s why we decided to leverage AI—offering each user an invisible, perfect assistant who can organize everything in their library and find exactly what they need in just seconds.
Long story short, AI algorithms can automatically tag and describe assets, saving you hours (or even days) of manual work. This not only speeds up the organization process but also reduces the errors that naturally come with human input. For example, tagging one asset as "United States" and another as "U.S." might not seem like a big deal, but when it’s time to search, those inconsistencies can lead to incomplete and frustrating results.
AI ensures consistency, meaning your searches will be more accurate and effective, even as your library continues to grow. Partnering with an AI development company can further enhances workflows by automating repetitive tasks and streamlining asset management, allowing your team to focus on more strategic work.
Here’s a quick breakdown of the main benefits of AI in DAM:
- Automated tagging and metadata management: AI handles the heavy lifting, tagging assets with relevant keywords and descriptions faster and more accurately.
- Image and video recognition: AI can identify objects, people, and even text within images and videos, making them searchable without manual input.
- Improved searchability and discoverability: With better metadata and organization, assets are easier to find, saving time and reducing frustration.
- Reduced manual labor and human error: AI minimizes the risk of inconsistent metadata and other common errors, ensuring more reliable data across your library.
- Optimization of workflows: By automating repetitive tasks, AI boosts efficiency and frees up your team for more meaningful work.
- Enhanced security and compliance: Some AI systems can even help with asset security, flagging sensitive data or ensuring compliance with privacy regulations.
- Time-saving: Ultimately, AI saves you hours—if not days—by automating tasks that would otherwise take up significant time.
In 2024, with AI becoming an integral part of DAM systems, it’s clear that the future of asset management is smarter, faster, and more efficient than ever.
AI Technologies Used in DAM
For the curious readers who want a peek inside the "artificial brain" of DAM, we’ve put together a simple and relatable breakdown of the main AI technologies behind digital asset management. We promise to keep it light—so if you're a tech pro, bear with us—we didn’t want to overwhelm anyone with too much jargon.
At Pics.io, we rely on a few key AI technologies that power our digital asset management system:
- Machine Learning (ML) – This is probably the most recognized type of AI. Think of ML as an algorithm that gets smarter over time. Developers train it on specific data sets, and from there, it learns patterns, makes predictions, and recognizes similarities. For example, show it enough images of different cat breeds, and it’ll eventually be able to tell a Siamese from a British Shorthair. In DAM, ML helps categorize and tag your assets by "learning" from the data.
- Natural Language Processing (NLP) – NLP is all about understanding human language. A great example of this is ChatGPT (yep, that’s me!). NLP models can read, interpret, and even generate human-like text. At Pics.io, we see the potential of combining NLP with image recognition to generate accurate, meaningful descriptions for your digital assets.
- Computer Vision – This is a game-changer for DAM. Computer vision allows AI to "see" and analyze the visual content of images and videos. It’s the technology that automatically tags and categorizes your media based on what’s inside the image. Whether it’s identifying a landscape or recognizing a product, computer vision does the heavy lifting for you.
- Deep Learning (DL) – Deep learning takes things up a notch. It uses neural networks with multiple layers (hence "deep") to understand complex patterns in large datasets. In DAM, DL helps refine tasks like keyword tagging and asset categorization, ensuring even your most intricate files are well-organized.
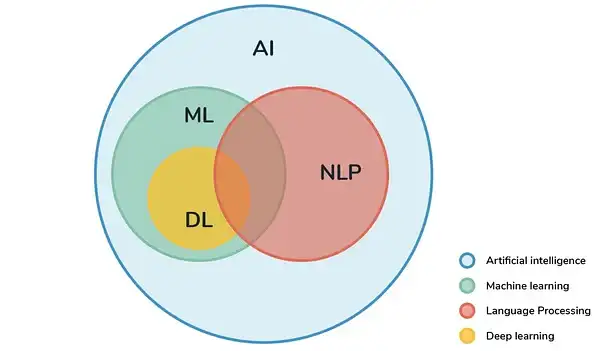
Examples of Successful AI Implementation in DAM
There are a lot of AI-powered tools developed by Top artificial intelligence companies on the market, but we’ll get to those later. For now, let’s take a closer look at the most popular features that Pics.io users love.
AI Keywording
This was one of the first AI features we implemented: AI-powered keyword generation, supported by computer vision technology. Keywords are the backbone of any advanced search in a DAM system, helping users quickly find assets. But when you’ve got a large library of assets, manually tagging each one with keywords can become a monumental task.
That’s where our AI comes in. Using advanced algorithms, Pics.io, one of the best project management software options available, allows you to bulk tag assets, drastically cutting down the time spent on metadata management.In fact, we recently upgraded our AI model, and the results are impressive: it now generates 63% more relevant keywords for images compared to the previous version.
What makes our AI stand out is its ability to provide context-specific tags. For instance, instead of generic tags like “machine” or “metal” for an image of someone rolling pasta dough, our AI now suggests far more accurate keywords like “rolling pin,” “chef,” and “flour”—tags that truly capture what’s happening in the image. This level of precision helps our users find exactly what they need, when they need it, making the search experience much more intuitive.
Face Recognition
Another powerful AI feature in Pics.io is facial recognition, which, despite concerns around data security in the tech world, has proven incredibly useful for certain industries. We’ve designed this feature with privacy in mind, ensuring the AI only works within your local DAM media library and doesn’t access any external databases.
Here’s how it works: when you upload images featuring people, our AI detects and highlights faces. You, the user, then assign a name to each face, and the DAM system remembers those names for future searches across your library. For example, if you tag one image with “John Doe,” the AI will automatically recognize John in other images and tag him accordingly. This makes searching by people a breeze, especially for industries like modeling agencies, media outlets, and press teams who frequently work with images of the same individuals.
It’s important to note that our system doesn’t "know" anything about the faces until you assign a name. Even if it detects a celebrity in your photos, it won’t identify them unless you add the tag yourself, keeping the environment secure and private.
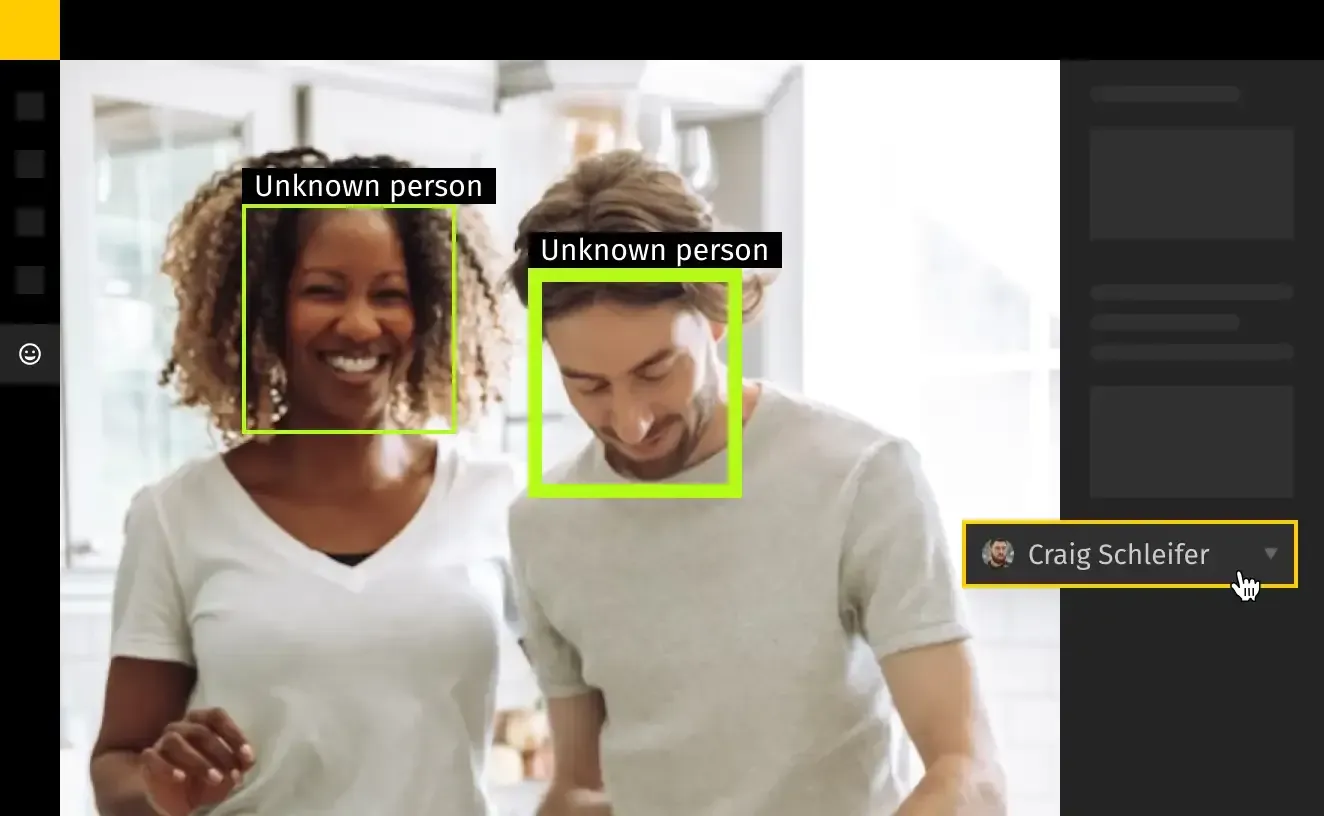
AI Visual Search
Another cutting-edge feature in Pics.io is AI visual search (also known as semantic search), which allows users to search for assets using more natural queries.
Our AI analyzes the visual elements of images to find similar ones in your library, so you don’t need to know the exact metadata to locate an asset. You can type something like "blue and yellow" or "cute kitten" into the search bar, and the system will offer you relevant assets with those visual elements.
Here’s why this is so important: sometimes, you don’t have the right words to describe what you’re looking for, or the asset might not be properly tagged. Visual search solves this by focusing on the actual content of the image—whether it’s shapes, colors, or objects. For instance, AI can identify images based on visual features like color gradients, light patterns, and composition. AI visual search simplifies the process, making it faster and easier to find the right image.
AI Speech-to-Text Transcription
Another key AI feature in Pics.io is AI speech-to-text transcription. This is especially valuable for managing video and audio files, where sorting through hours of content to find specific information can be a challenge. With AI speech-to-text, spoken content is automatically transcribed, making it searchable and easy to navigate.
If you have a video interview or a podcast recording, instead of manually scrubbing through the entire file to locate a key section, you can search for specific keywords in the transcript, and the AI will take you directly to the relevant part of the video or audio. This feature not only saves time but also enhances accessibility, allowing teams to quickly extract and repurpose content.
For industries like media production, education, or any business that deals with large volumes of video and audio content, AI transcription is a game-changer. It ensures that valuable insights or important moments in your recordings are easily accessible, reducing the time spent on manual review.
Best Practices for Implementing AI in DAM
AI is transforming DAM systems, no doubt about that. It’s packed with features that streamline workflows and boost efficiency, but let’s be honest—AI also raises costs. It might seem odd at first: shouldn’t AI make things cheaper, not more expensive? But remember, AI is a resource-heavy technology. It requires a lot of processing power, and that comes with a price. So, while AI tools offer incredible advantages, the best practice is to figure out what your team really needs before diving in.
Let’s take a quick look at some of the additional AI tools available in DAM, beyond the features we’ve already mentioned, and what they can do for you:
- Content recommendations: Suggests assets with similar themes, helping you quickly find relevant files and streamline your workflow. Just keep in mind, if the AI isn’t trained well, it might suggest irrelevant content.
- DAM analytics and insights: Gives you detailed insights into asset usage and performance, which can help optimize your content strategies. You might need to integrate other analytics tools for deeper insights.
- Cropping and editing: Automatically crops, resizes, and enhances images, saving you time on manual editing. Just note that the quality may vary based on the complexity of the task.
- Similarity detection: Finds visually similar assets, helping you quickly locate related files. It works well but might struggle with abstract content.
- Duplicate detection: Identifies and removes duplicate files, preventing storage clutter. Be cautious, though—false positives can sometimes cause the deletion of important assets.
- Color recognition: Categorizes assets based on detected colors, which is helpful for branding projects. However, color detection doesn’t always match user expectations.
- Landmark detection: Identifies famous landmarks in images and videos, helping you organize location-based content. Accuracy can vary depending on the recognition model.
- Content creation: Allows DAMs to also serve as content creation platforms, generating and editing assets. Content quality depends on the AI’s training.
- Character recognition (OCR): Extracts and indexes text from images or PDFs, making them searchable. It’s a time-saver, but it can struggle with poor image quality or complex fonts.Solutions like OCR Studio provide robust, on-premise OCR capabilities that help overcome these challenges with high accuracy and security.
- Object recognition: Tags objects in images, reducing manual work and speeding up asset organization. Accuracy varies with more complex objects.
So, are AI-powered tools the right fit for you?
Before jumping in, it’s crucial to factor this into your budget and figure out whether AI tools will truly add value to your DAM system.
Here’s a quick step-by-step guide to help you figure it out:
- Identify use cases: What do you need AI for? If you’ve recently migrated thousands of assets into your DAM and they’re missing metadata, AI could be a huge help. Or maybe you run a talent agency and need to quickly access photos of your clients. In those cases, AI could be a game-changer.
- Audit your library: Take a good look at your assets. How many do you have, and how many need AI processing? Will AI genuinely help in a meaningful way, or could some tasks be better handled manually? If your needs are so specific that AI might struggle, it could end up being more hassle than help.
- Do a test run: Before going all in, test AI on a small batch of assets. Whether you use your existing account or take advantage of a free trial, this test will show you how well AI handles your tasks. It’s the best way to work out any potential kinks and see if AI is worth the investment.
Let’s face it: AI can do amazing things, but it’s not a one-size-fits-all solution. The key is understanding what your team really needs and making sure that AI will bring value to your DAM workflow. We’ve seen firsthand how transformative it can be at Pics.io, but we’ve also learned that careful implementation is essential to getting the most out of it.
Wrapping up: Future Trends of AI in DAM
The widespread implementation of AI technology is set to revolutionize industries across the board, unlocking new possibilities in countless areas. As we look to the future, it's clear that AI will continue to play a major role in shaping digital asset management. We’re already seeing AI streamline workflows, automate tedious tasks, and help teams work more efficiently—but the real breakthroughs are still to come.
We expect AI to become even smarter and more intuitive. Imagine better content recommendations, more accurate search results, and advanced personalization that adapts DAM systems to how you and your team work best. We’re also excited about the potential for deeper integrations with other tools, creating an ecosystem where everything seamlessly connects. And let’s not forget, AI’s capabilities in handling video and audio content will only get better, making it easier than ever to organize and utilize diverse digital assets.
That said, balance is key. While AI can handle a lot of the heavy lifting, it’s important to ensure you’re using the right tools for the right tasks. As AI continues to evolve, the trick is staying curious and adaptable—knowing when to rely on AI and when a human touch is still the best approach.
Did you enjoy this article? Give Pics.io a try — or book a demo with us, and we'll be happy to answer any of your questions.